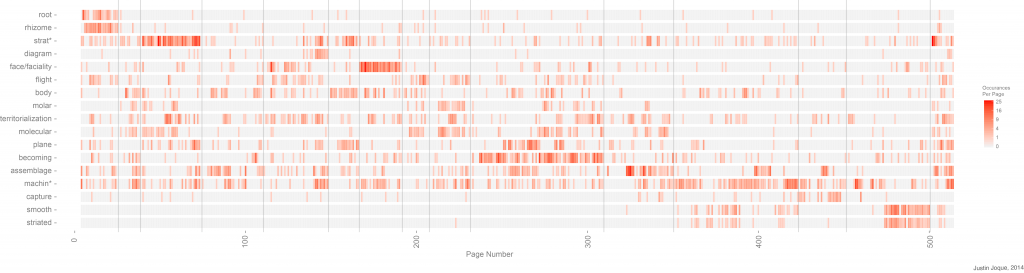
Gilles Deleuze and Felix Guattari’s “A Thousand Plateaus” is, arguably, the duo’s most important work, fleshing out important concepts like the rhizome in the 600+ page tome. It also utilizes a non-linear writing style that casually injects phrases like “God is a lobster” into some of the most provoking philosophical thought of the 20th century.
Now, a grad student at the European Graduate School, Justin Joque, has decided to map the duo’s language. Any work on Deleuze and Guattari are rife with terms like “assemblage,” “molar,” “becoming” and so on. The map illustrates the jargon as it appears, disappears, and reappears in the text.
“The red bars represent the number of occurrences of key phrases that appear, build and disappear throughout the text. The vertical grey lines mark the given division of the text into sections,” Joque writes of his method. The 1987 English translation was used for analysis.
Deleuze and Guattari’s (quoted by Joque) describe their work in “A Thousand Plateaus”:
We are writing this book as a rhizome. It is composed of plateaus. We have given it a circular form, but only for laughs. Each morning we would wake up, and each of us would ask himself what plateau he was going to tackle, writing five lines here, ten there. We had hallucinatory experiences, we watched lines leave one plateau and proceed to another like columns of tiny ants. We made circles of convergence. Each plateau can be read starting anywhere and can be related to any other plateau (22)
Check out Justin Joque’s page here.